

The Medical Data Analytics Laboratory (MeDA Lab) at National Taiwan University aims at building a world-leading platform for Artificial Intelligence for Medical Image Analysis (AIMIA Platform). The Platform consists of a high-performance Artificial Intelligence Engine (AI Engine) and innovative Augmented Intelligence Workflows (AI Workflows). MeDA Lab establishes the platform with comprehensive knowledge of artificial intelligence, medicine, high-performance computing, mathematics, and statistics. We put together talents and resources from world-renowned universities and enterprises; we connect international and interdisciplinary researchers and professionals; we collaborate with the public sectors like the Taiwanese National Health Insurance Administration. As our motto goes, “help doctors to help people”, MeDA Lab is determined to develop the AIMIA Platform as clinicians’ best AI assistant and help medical personnel tackle the upcoming challenges of precision medicine.
Applications
Clinical workflows in healthcare
Advantages
The AIMIA Platform by MeDA Lab will offer a total solution for medical image analysis and its applications in medical care, with solid scientific evidence. AIMIA includes two tracks: the AI Engine and AI Workflows. The former extracts hidden information from high-dimensional medical image data sets. The latter turns the information into clinical intelligence. The AIMIA Platform combines functions that can be coordinated according to different medical workflows in order to launch customized medical assistance systems that embrace both commonalities and variations.
Related Links
Lab Website: http://meda.ai
Keywords
Artificial intelligence, medical image analysis, algorithm and software modules, clinical medical care workflows
◎ PI
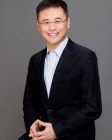
PI Weichung Wang
Professor, Graduate Institute of Applied Mathematical Sciences, NTU
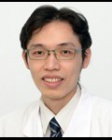
Co-PI Chia-Chun Wang
Attending Physician, Department of Oncology, NTUH
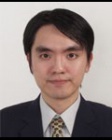
Co-PI Che-Yu Hsu
Attending Physician, Department of Oncology, NTUH

Co-PI Ting-Li Chen
Associate Research Fellow, Institute of Statistical Science, Academia Sinica
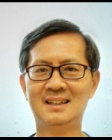
Co-PI Mao-Pei Tsui
Professor, Department of Mathematics, NTU
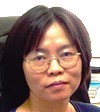
Co-PI Su-Yun Chen
Research Fellow, Institute of Statistical Science, Academia Sinica
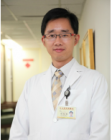
Co-PI Chih Chieh Yang
Attending Psychiatrist, Department of Psychiatry, Taipei Veterans General Hospital
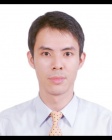
Co-PI Wei-Chih Liao
Associate Professor, Department of Internal Medicine, College of Medicine, NTU
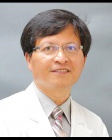
Co-PI Shih-Jen Tsai
Chief, Department of Psychiatry, Taipei Veterans General Hospital
[2020/06/01] Research paper "Deep learning to distinguish pancreatic cancer tissue from non-cancerous pancreatic tissue: a retrospective study with cross-racial external validation" released on The Lancet Digital Health.
Link to the paper: https://www.thelancet.com/journals/landig/article/PIIS2589-7500(20)30078-9/fulltext
"PANCREASaver" introduction:http://pancreasaver.ai
MeDA Lab introduction: http://meda.ai
Background
The diagnostic performance of CT for pancreatic cancer is interpreter-dependent, and approximately 40% of tumours smaller than 2 cm evade detection. Convolutional neural networks (CNNs) have shown promise in image analysis, but the networks' potential for pancreatic cancer detection and diagnosis is unclear. We aimed to investigate whether CNN could distinguish individuals with and without pancreatic cancer on CT, compared with radiologist interpretation.
Link to the paper: https://www.thelancet.com/journals/landig/article/PIIS2589-7500(20)30078-9/fulltext
"PANCREASaver" introduction:http://pancreasaver.ai
MeDA Lab introduction: http://meda.ai
Background
The diagnostic performance of CT for pancreatic cancer is interpreter-dependent, and approximately 40% of tumours smaller than 2 cm evade detection. Convolutional neural networks (CNNs) have shown promise in image analysis, but the networks' potential for pancreatic cancer detection and diagnosis is unclear. We aimed to investigate whether CNN could distinguish individuals with and without pancreatic cancer on CT, compared with radiologist interpretation.
Methods
In this retrospective, diagnostic study, contrast-enhanced CT images of 370 patients with pancreatic cancer and 320 controls from a Taiwanese centre were manually labelled and randomly divided for training and validation (295 patients with pancreatic cancer and 256 controls) and testing (75 patients with pancreatic cancer and 64 controls; local test set 1). Images were preprocessed into patches, and a CNN was trained to classify patches as cancerous or non-cancerous. Individuals were classified as with or without pancreatic cancer on the basis of the proportion of patches diagnosed as cancerous by the CNN, using a cutoff determined using the training and validation set. The CNN was further tested with another local test set (101 patients with pancreatic cancers and 88 controls; local test set 2) and a US dataset (281 pancreatic cancers and 82 controls). Radiologist reports of pancreatic cancer images in the local test sets were retrieved for comparison.
Findings
Between Jan 1, 2006, and Dec 31, 2018, we obtained CT images. In local test set 1, CNN-based analysis had a sensitivity of 0·973, specificity of 1·000, and accuracy of 0·986 (area under the curve [AUC] 0·997 (95% CI 0·992–1·000). In local test set 2, CNN-based analysis had a sensitivity of 0·990, specificity of 0·989, and accuracy of 0·989 (AUC 0·999 [0·998–1·000]). In the US test set, CNN-based analysis had a sensitivity of 0·790, specificity of 0·976, and accuracy of 0·832 (AUC 0·920 [0·891–0·948)]. CNN-based analysis achieved higher sensitivity than radiologists did (0·983 vs 0·929, difference 0·054 [95% CI 0·011–0·098]; p=0·014) in the two local test sets combined. CNN missed three (1·7%) of 176 pancreatic cancers (1·1–1·2 cm). Radiologists missed 12 (7%) of 168 pancreatic cancers (1·0–3·3 cm), of which 11 (92%) were correctly classified using CNN. The sensitivity of CNN for tumours smaller than 2 cm was 92·1% in the local test sets and 63·1% in the US test set.




